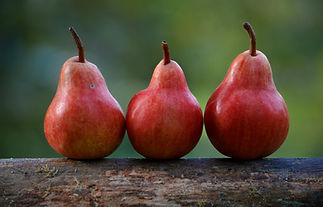
Caliper matching
Propensity score matching is a very popular method to perform causal inference. There are many ways to create matches. In this project, we investigated the asymptotic properties of propensity score matching with a caliper.
Summary
Propensity score matching is a tremendously popular method used to draw causal conclusions from observational data. People with different treatment assignments are matched if they are similar in terms of characteristics that are related both to treatment and the outcome. For example, if someone would like to investigate whether a vegetarian diet causes a longer life, they would look for people with and without a vegetarian diet and match them on factors like age, sex, social economic status, smoking habits, exercise habits and so on. If there are many important factors, it quickly becomes impractical to find exact matches on every aspect. Instead, as shown by Rosenbaum and Rubin in 1983, it is sufficient to make matches based on the propensity score: the probability of getting a particular treatment, given each person's relevant characteristics.
There are many ways to make matches based on propensity scores. We studied caliper matching, which works as follows. First you select the caliper, a typically small number. For example, let's say we set the caliper at 0.02. Then we consider someone who is in one treatment group and look for matches in the other treatment group. We consider them a match if the difference between their propensity scores is at most the caliper. For example, if we have a vegetarian with a propensity score of 0.74 and we used a caliper of 0.02, we would match that person to all non-vegetarians with a propensity score between 0.72 and 0.76.
An important question is how to choose the caliper. Should it be 0.02, or maybe 0.01 or 0.05 or something else? With a larger caliper you get more matches, but there is a risk that matches will not be similar enough anymore. With PhD student Máté Kormos and Aad van der Vaart, we investigated the asymptotic properties of caliper matching. We proved that the resulting estimator of the average treatment effect, and average treatment effect on the treated, is asymptotically unbiased and normal at parametric rate. From this analysis we derived recommendations for setting the caliper in practice.
Related papers
Kormos, M., van der Pas, S., van der Vaart, A. (2023). Asymptotics of caliper matching estimators for average treatment effects. arXiv:2304.08373 [link]